Practical implementation – KI Reallabor
All further explanations in the field of artificial intelligence are preceded by the fact that – contrary to many market offers – there is no “AI box” with the help of which artificial intelligence can be implemented in one’s own company with the greatest of ease. In our more than 15 years of research and experience in practical implementations of technologies like pattern recognition, machine learning and others, which are summarized under the term “artificial intelligence”, we can only recommend a level-headed as well as well thought-out and planned implementation. A realistic and step-by-step assessment and implementation of suitable technologies for each individual case is today’s reality, because the current possibilities are manifold and complex. In order to fully develop the technological potential, we are happy to offer you professional support in the orientation and selection of suitable technologies. For this purpose the AI Reallaboratory is a suitable and free platform to find this support and orientation and to place problems. Information about the AI Reallaboratory can be found on our website.
Machine learning
Data analysis and process modelling technologies are increasingly used for early fault detection, prediction of machine behaviour and maintenance as well as for more efficient operation. A step-by-step integration of machine learning technologies makes sense for most companies taking into account the degree of automation.
1. feasibility studies
An analysis of the available data from technical systems and an estimation of the potential for the use of ML in production operations will precede. Based on the first results
a preparation and visualization of the data as well as the use of different ML methods for model identification to answer basic questions can be defined.
2. process data analysis
Based on the provided data, an application of different ML-methods for offline analysis can be implemented, taking into account special issues such as detection of outliers or a cause of error, determination of optimization potential, analysis of cycle times, etc.
3. real-time plant monitoring
Integration of ML methods into the online operation of the plant in order to detect errors during operation, as well as early detection of signs of wear (predictive maintenance).
Contact
Ansprechpartner:
Dr. Alexander Maier
Fraunhofer IOSB-INA
Campusallee 1 | 32657 Lemgo
Engineering
Efficiency-increasing consistency in engineering is not possible today due to a large number of breaks in the engineering process. These breaks have different origins: missing data interfaces between engineering tools prevent the direct reuse of development results from other domains (e.g. mechanics, electrics, software).
An approach to achieve consistency in engineering is based on a data model that underlies all the trades. Each domain can write into this model with its specific engineering tools, or extract existing data from this model. Another important aspect in this context is the consistency in service-oriented architectures. Many efforts are currently focused on the digital description and standardization of capabilities. Capabilities correspond to abstract or concrete automation functions that are required for the product manufacturing process. In order to achieve consistency in this area as well, however, it is also necessary to standardize the description of the product to be produced. This enables, for example, an automatic matching between the product description and the description of capabilities of a machine, a machining station or individual components.
This is a basic requirement for automated system planning and/or process orchestration for modular and distributed production systems.
Contact
Ansprechpartner:
André Mankowski, Philip Priss,
Natalia Moriz
Technische Hochschule OWL
Campusallee 6 | 32657 Lemgo
Sensor orchestration and information fusion
It is a continuing trend that sensors are produced at ever lower costs. Industrial systems such as production lines, process plants, but also cars benefit both from measurement technology that was too expensive just a few years ago and from the redundancy of multiple sensors. Traditionally developed solutions, however, are not able to exploit the full potential of these emerging multi-sensor systems. Sophisticated concepts for information fusion make multisensory data processing possible. Automated sensor orchestration enables highly complex application scenarios for multi-sensor systems. Information fusion and sensor orchestration are current research topics at the Institute of Industrial Information Technology.
Contact
Ansprechpartner:
Prof. Dr. Ing. Volker Lohweg
inIT | Technische Hochschule OWL
Campusallee 6 | 32657 Lemgo
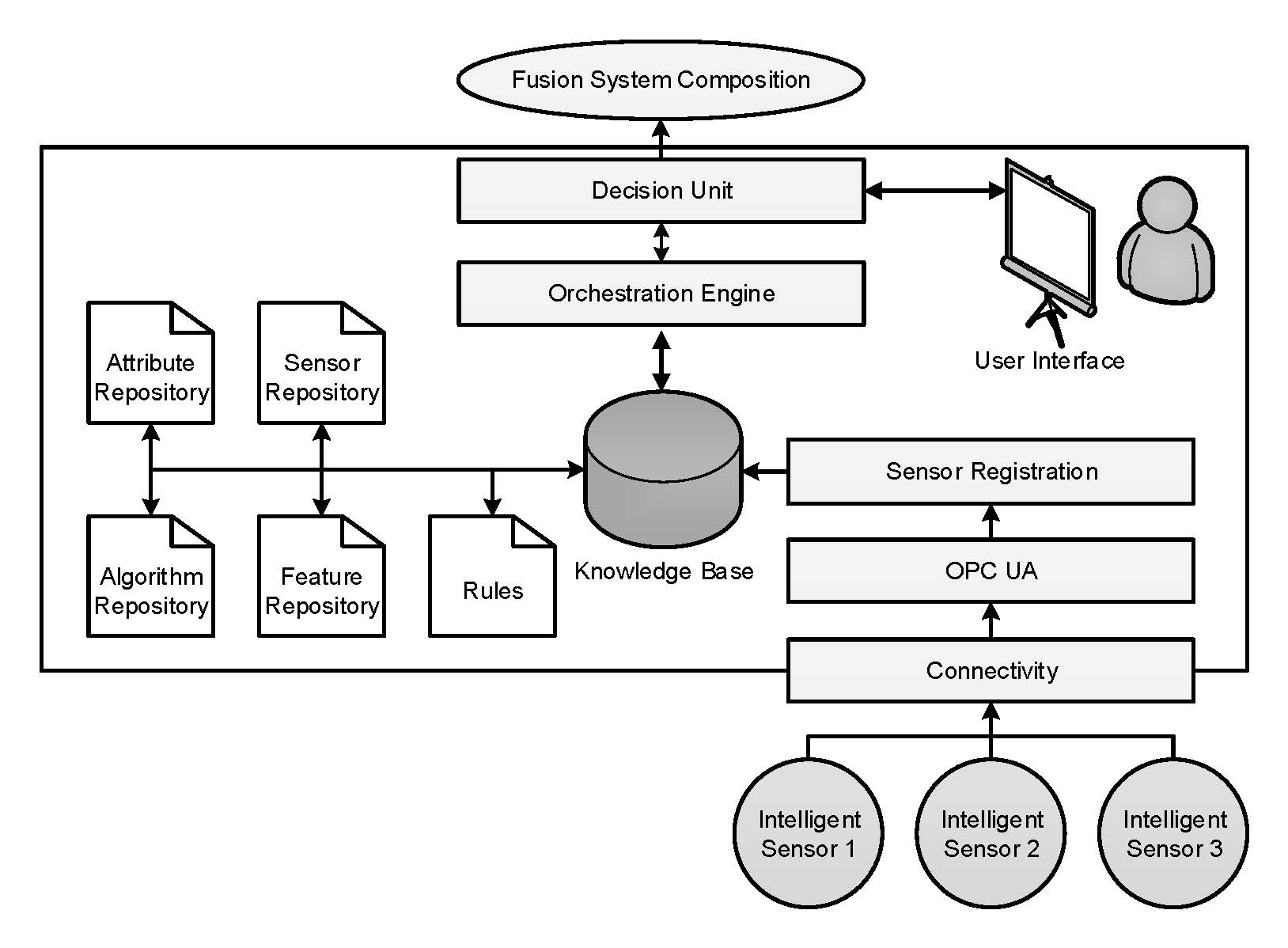