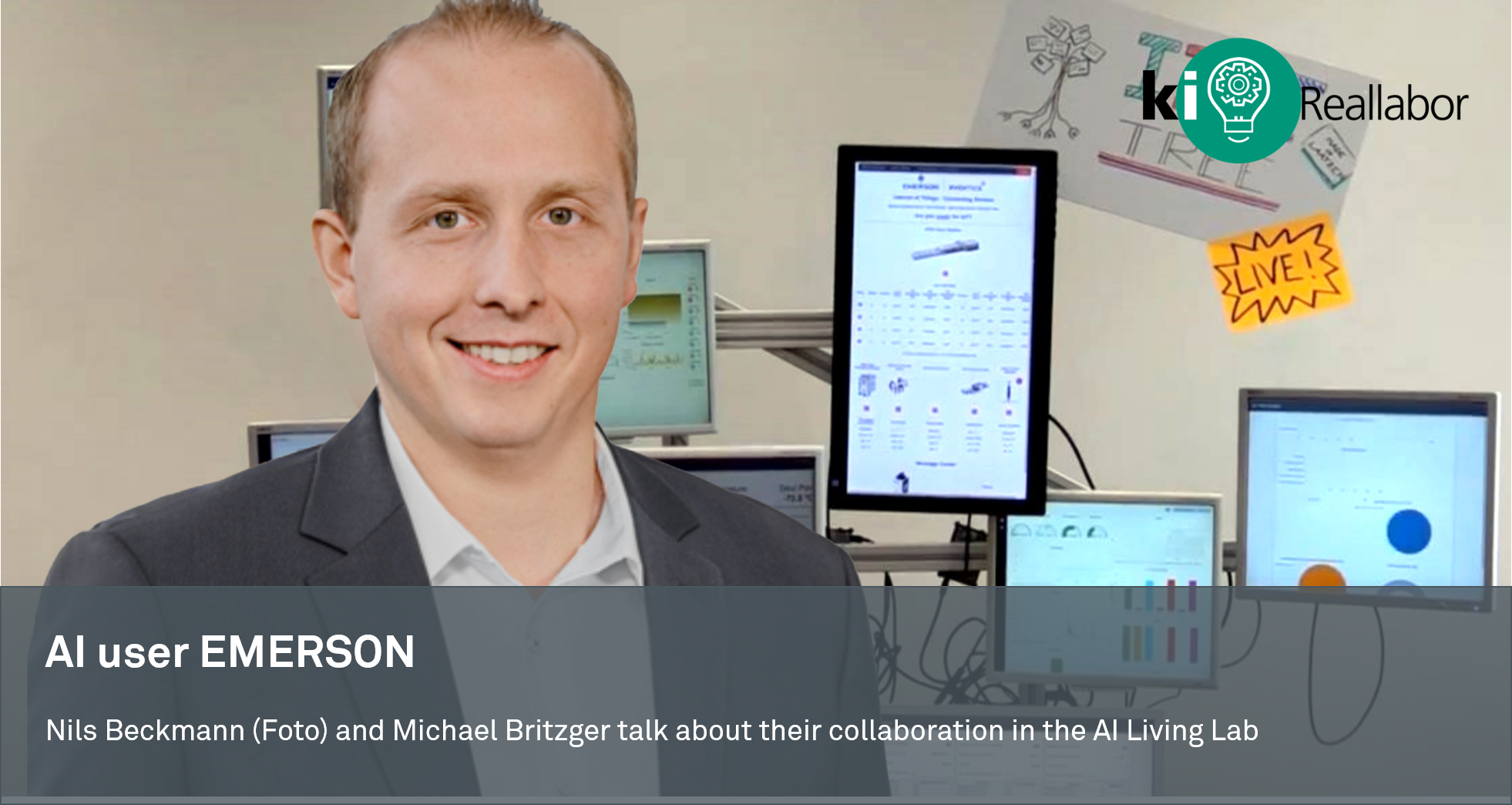
Intelligent compressed air control and leakage detection
The energy supply in a company is not only essential for the successful, industrial manufacture of products, it also represents a considerable cost factor in operation. This makes it all the more important for both manufacturing companies and manufacturers of energy supply components to focus on optimizing their production and products.
This is also the case with Emerson Aventics – a leading global manufacturer of pneumatic air valves. These are used in particular to drive mechanical systems such as conveyor belts and cylinders, for example in injection molding machines. For some time, Emerson has been researching and developing solutions for faster and more accurate detection of leaks in the compressed air supply to production systems. When such leaks occur, compressed air escapes continuously or intermittently from a supply system, resulting in insufficient compressed air arriving at the desired process. Ergo, the system has to produce more compressed air and consumes unnecessary energy. Since a drop in compressed air is not very noticeable – usually only in the ratio of pressure produced from the air compressor and pressure arriving at the desired process – it is very complex to locate the leakage.
Emerson Aventics approached the AI Living Lab in Lemgo with this challenge. The data set provided by Emerson included tabulated data from a test setup in which leakages and information about them were specified. Ideally, an algorithm was to be developed in the AI Living Lab to machine-detect where these leakages occur and whether there are patterns. The AI Living Lab provided support in preparing the data and designing the tasks and organized a challenge together with the AICommunityOWL to familiarize as many AI experts as possible with this task.
“Digitization is an immensely important component for success, or more precisely, it is even the foundation. The mindset of people, in turn, forms the basis for digitization, which then makes being successful possible. At Emerson, we have dealt with precisely this mindset and want to move forward innovatively. This is precisely why we decided to participate as a challenge donor and open up our mindset in even more directions,” describes Michael Britzger from Emerson.
Based on the test data, several teams developed proposals using a machine learning method. The first results could be seen after just a few days. Now, one of the teams that showed a promising approach will continue with an implementation in a real production setup at SmartFactoryOWL. Here, an unsupervised learning approach will be tested over the course of the project, which should make it possible to detect and localize leaks without prior knowledge of the algorithm.
Michael Britzger is enthusiastic about the momentum and is already looking to the future: “Really remarkable results have emerged, and we now want to build on them. This is definitely not the end of the story.”